Internet of Things in Healthcare
New Opportunities, Challenges, Case Studies, and Applications of Edge AI for Connected Healthcare
Introduction
Healthcare is a very crucial aspect of human life. Because of the rapid growth in population and the rise of various diseases, there is also a drastic surge in the demand for healthcare and related facilities. In addition to this, the evolution of technology and the emergence of the Internet of Things (IoT), AI combined with advancing next-generation wireless communications, the notion of connected or smart healthcare has now been introduced to be a developed version of the traditional systems of healthcare. As a matter of fact, smart healthcare can be rendered as that health service that utilizes advanced technologies such as the Internet of Things (IoT), wearable devices along with advanced communication protocols so as to connect patients, the caregivers as well as healthcare institutions in a dynamic way so that information can be transformed easily among them [1].
Healthcare services which are categorized as smart healthcare services have made the possibility of handling as well as responding to different types of medical requests distantly in an intellectual manner hence, reducing the factor of hospitalization to a greater extent along with helping healthcare providers and other people to detect, predict, diagnose as well as treat the diseases in an intelligent way. In addition to this, smart healthcare e-services are also very useful in the prevention and controlling of the outbreak of infectious or contagious diseases like avian influenza, Ebola virus, Chickungunya virus [2], and the recent Covid-19 pandemic. With all these pieces of information in hand, it has become empirical that the adoption of smart healthcare services is the best way to improve the health level of any country.
An increasing amount of interest is being developed towards those architectures which tend to realize the cooperation of Fog, Cloud, and Edge computing in the healthcare sector. The primary aim is to exploit the potential of edge nodes so as to not only handle functional tasks but, also in data processing, analysis, inference, and correlation [3].
These approaches tend to make the future of the implementation of reliable distributed healthcare services and applications promising as the intelligent mapping of resource management and computational tasks across the nodes proves to fulfill the stringent requirements of the Internet of Things in the healthcare sector [4].
Within this context, the spread of ‘Edge/Fog Health’ solutions which uses appropriate computing models so as to distribute health sensors data processing as well as storage amongst various nodes that are situated at different levels of user proximity, as follows:
Edge computing tends to incur directly over devices to which the sensors are connected or any gateway device which is physically proximate to the sensors. Some good examples of edge nodes include wearable devices like smartphones.
Fog computing nodes are the local area network so that bigger and powerful devices like gateways, local servers, and PCs can be included which might be at a distance physically from the actuators and sensors.
Both of these paradigms which are increasingly being implemented in a combined manner tend to leverage the proximity of the users so as to deliver location-aware healthcare services with lower latency and higher availability [5]. Within these dimensions, some methods rely on hierarchical computing strategies that were proposed to allocate as well as distribute the inference tasks of Artificial Intelligence (AI) and Machine Learning (ML) methods between the fog, cloud, and edge levels attempting to push computational capacities of the edge devices to their top [1], [4], [6] and [7].
A transition from the mobile cloud computing model (MCC) which is characterized by increased data transmission costs as well as limited coverage towards a mobile edge computing model (MEC) [8] with lower latency and reliable edge ML approaches has been progressively been made part within the smart healthcare realm.
Edge Technology in Smart Healthcare
In recent times, most healthcare systems have implemented cloud computing solutions for the provision of accessible and affordable healthcare solutions so as to process as well as store large amounts of data that have been recorded through various biosensors. However, as cloud-based smart healthcare system usually involves a network, cloud servers, and mobile devices, there are many times a long distance amongst systems’ unit which, further strengthens the issue of higher latency within these systems [9]. Hence, real-time and emergency medical treatments cannot completely rely on cloud-based healthcare systems. In addition to this, the huge amount of information that is produced by these sensors should regularly be transferred over to the cloud so as to process and store information which can result in increased consumption of energy and increased cost as well. Moreover, many patients requiring treatment for chronic diseases require lower-cost mobile environments which lack support from cloud-based healthcare solutions [10] and [11].
Edge-assisted healthcare solutions transfer processes to the end-user which not only reduces the use of energy but also reduces response time. As a matter of fact, edge technology assists smart healthcare systems to mine and then process health information collected through edge services and devices that are near to the user. Additionally, processing information with edge also helps in boosting security, mobility, privacy, geographical distribution, lower network bandwidth use as well as location awareness along with facilitating on the web diagnosis and analytics thereby, reducing hospital visits [2]. Therefore, edge technology greatly contributes towards the development of a smart healthcare systems by delivering swift, comprehensive as well as universal treatments.
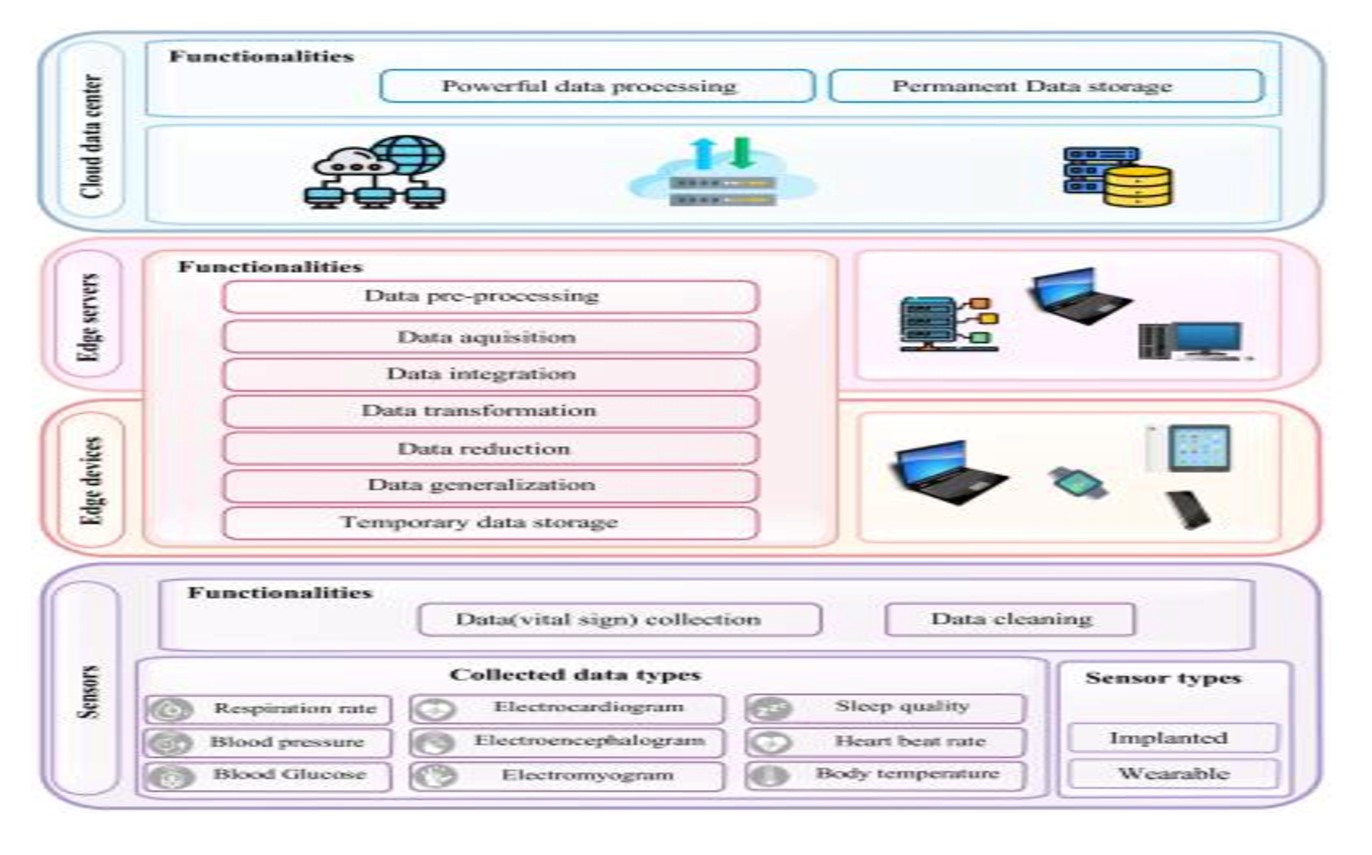
Fig. 1: Edge-assisted smart healthcare functionality flow.
The architecture of edge computing in smart healthcare services comprises four distinct layers including sensor layers, edge devices, edge servers, and cloud data centers. This architecture has been illustrated in Figure 1 in the form of functionality flow, representing the functions as well as the common devices that are being used at every layer. In the sensor layer, there are numerous sensors including both implanted as well as wearable sensors which have the function of obtaining health data and vital signs from the patients. The figure indicates the type of data which can be collected with the help of these sensors. This layer initiates communication with edge devices layer by means of low power and short-range wireless communicating protocols like Bluetooth, ZigBee, and Wi-Fi [10]. This layer includes users’ devices like smartphones, smartwatches, tablets, and raspberry pi which have the capability of conducting various processes as well as storing data in the shorter term.
The information in the edge devices layer is transmitted to the edge server layer through Wi-Fi, Bluetooth, or internet protocols. In the edge server layer, there are microdata centers through which it conducts numerous processes and stores more data against the edge devices layer [11]. Figure 1 illustrates that the functionality of the edge devices layer and edge servers’ layer is nearly the same except that the computational power and storage capacity in the edge server layer is a lot more powerful than that of the edge devices layer. The final layer of the cloud data center incorporates highly powerful processors and has the capability to store huge amounts of data. This layer develops communication with edge servers’ layer over the internet.
Artificial Intelligence in Edge Assisted Smart Health Care Systems
Artificial Intelligence (AI) has excelled in numerous sectors which have ultimately opened up a vast amount of possibilities for machines to start ‘thinking’ and automate tasks given which was once the duty of humans so as to gain faster and more accurate results. In recent times, AI has penetrated itself in the medical sector as well. With the combination of edge AI with reliable and strong computer hardware, medical technology is now capable of incorporating deep learning so as to deliver better and improved patient care as well as medical outcomes of the patients.

Internet of Things (IoT) and Big Data have emerged as major players in the healthcare sector. In the future, this sector can highly benefit through AI assistance or might even take over some tasks that are currently assigned to doctors and healthcare staff a good example of this is a radiologist have a task to analyze ultrasounds so as to arrive at a diagnosis of the patients. However, radiologists can assess only a few of the medical images at a time. When this task of analysis is allocated to AI software, its computer hardware building block will have the capacity to analyze multiple images at a very swift pace. In addition to this, the vast amount of historical data stored within the AI server, the system will also be able to identify any abnormalities along with calculating results on its own. Another benefit from these systems is that they can also offer customized treatment plans based upon the medical history in the patients’ records.
When the AI technology in the healthcare sector is attained at a perfect pace, the sector will be more capable of improving and saving lives along with reducing costs. Additionally, the technology will also eliminate the risk of any human error within the diagnosis and provide accurate and reliable information which is crucial for expedited care and medical treatment. This technology also fosters clinical workflow and free up a lot of time of doctors and physicians which can be used for patient interaction and consultation along with reducing the time involved in receiving treatment.
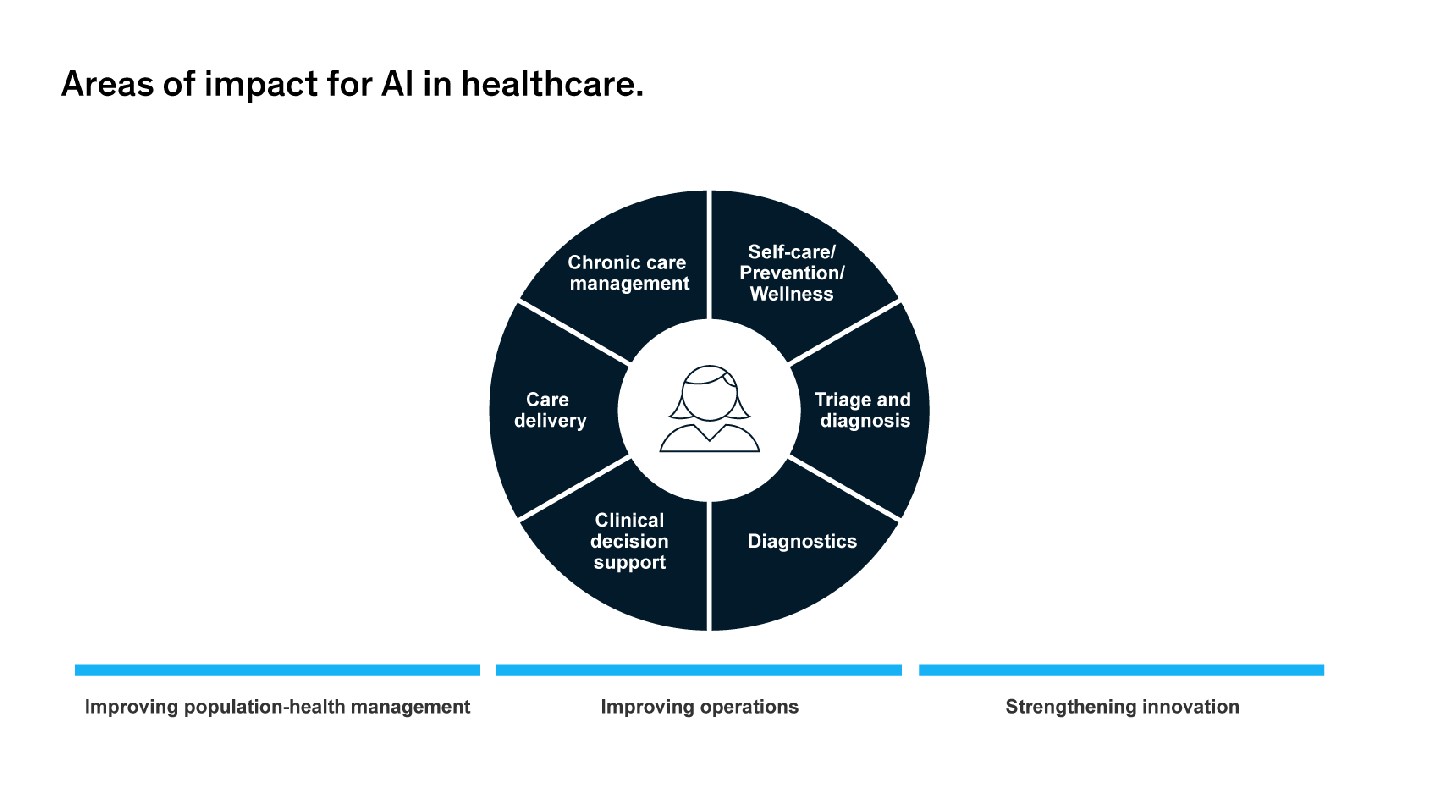
Opportunities and Challenges
Healthcare is at the edge of a revolution – one which is driven by technologies such as artificial intelligence (AI) and the other one with edge computing. The implementation of artificial intelligence in healthcare is expected to grow at an annual growth rate of 41.4% from 2020 to reach $51.3 billion by 2027, while edge cloud computing is expected to grow by 34.1% between now and 2025. As both technologies are growing continuously, they are greatly being made part of the decisions made in the healthcare sector. The technologies go hand in hand – AI has become the key driver for edge computing and edge computing is a significant enable for AI.
Read More:
IoT-based Smart Campus: An exploratory study of the effect of administration, instructors and student’s awareness levels on adopting IoT-based Smart Campus Chapter 1
Transforming the rate of biomedical discoveries through Edge-AI
In this era of the COVID-19 pandemic, the healthcare sector has transformed towards AI for accelerating research over COVID-19 so as to discover more about the virus as well as being able to develop suitable antiviral medicines. A UK-based technological organization, Causaly, developed a biomedical research tool that allows researchers to carry out deep researches and find answers to complex research questions which would take weeks and even months otherwise. The AI of Causally can read, understand as well as interpret a huge database of biomedical information within seconds, allowing researchers to rapidly map epidemiology data, molecular targets, biomarker genes along with identifying potential treatment options [12].
These types of AI applications are traditionally powered by cloud computing and data centers. However, with time AI has become a lot closer to the user – within software and Internet of Medical Things (IoMT) endpoints as well as other medical devices. Examples can be wearable health devices like blood pressure monitors and ECG monitors which can collect and assess data locally and which can be easily shared by a patient to the doctor for carrying out an instant health assessment.
The result of this is that more and more healthcare businesses that are involved in AI have started to understand the importance of edge computing. As a matter of fact, Deloitte expects to sell more than 750 million edge AI chips which have been designed to allow on-device machine learning this year.
Transferring the Dull Work from Doctors to Machines
The use of edge-AI algorithms to support medical decision making, workflow management, and early diagnosis is relevant because the technology does not suffer from human deficits such as fatigue, its use would result in care more efficient for patients and time savings for healthcare professionals.
An average nurse in the US spends almost 25% of her time on administrative tasks. This can easily be reduced by the implementation of Robotics Process Automation. Moreover, Natural Language Processing (NLP)-based assistants like chatbots used to perform initial patient interviews are an off-the-top-of-the-head example. Developing a comprehensive Electronic Health Record System using edge computing technologies and powered by AI is the first step towards the development of the next generation Edge-AI powered healthcare solutions [13].
Administrative Workflow Management: Using edge-AI to automate administrative workflow through custom software development allows physicians to save time on routine tasks and prioritize issues urgent. Managing routine tasks, such as entering medical notes into patient charts, can be done using audio-to-text transcription software, saving valuable time.
The use of chatbots coupled with technology can also save caregivers precious time. The sculpin is able to communicate with patients, in order to follow them at home and trigger an alert in the event of an abnormal response. The caregiver can then take over, and devote his time to the patients for the patients who really need it [14].
Predictive analysis: Patient data collected from medical records and data obtained from connected objects give the doctor access to valuable information on the patient as well as on the population cohort to which the patient belongs. Computing this data using AI algorithms and edge computing helps develop the patient profile and build predictive models to effectively anticipate, diagnose and treat disease.
Prognosis: The massive use of data by AI makes it possible to improve the prognosis of patients by adapting the treatment to the characteristics of the disease and the specificities of each person. This precision medicine thanks to edge computing now offers the possibility of prescribing the best therapeutic options according to a very particular profile to maximize the chances of success of the treatment [15].
Providing Early Diagnostic Services
The proliferation of connected objects combined with the computing power of Edge-AI has enabled healthcare professionals to better monitor the patient and detect life-threatening risks at an earlier stage and easier to treat [16]. Some examples of applications of this data that are currently used in the health sector
Heart Condition Detection: Connected devices can be used to track the heart rate and monitor the patient’s EKG. This allows underlying heart conditions to be detected and diagnosed earlier. This is the case of the European MAESTRIA project, the objective of which is to prevent heart rhythm disorders and the risk of stroke, by integrating artificial intelligence. The mass of data from different medical sources can thus be analyzed in order to offer personalized therapies to each patient [17].
Breast cancer detection: AI is currently used to analyze mammograms. It was discovered that the scan rate is 30 times faster than that of a human and has an accuracy of 99%. It is able to detect extremely small metastases that humans are likely to miss. This not only reduces the risk of misdiagnosis but also reduces the need for invasive biopsies to make the diagnosis. This is the case with the company Mammoscreen, which uses AI and edge computing for the interpretation of screening mammograms [6].
Disease Trends: Patients are increasingly relying on search engines like Google to check their symptoms online before going to the doctor. Using AI to monitor this trend and draw conclusions can lead to early intervention in the event of a possible outbreak in the population. Google had already tried to do this in 2008 with influenza trends with its Google Flu Trends tool, but it failed due to the lack of streamlined data and numerous inconsistencies [18].
With advances in computing, this can now become an important asset for the early detection of infectious diseases and the prevention of their epidemics. From the end of December 2019, the Canadian company BlueDot had alerted to the coronavirus epidemic, even before the first alert from the World Health Organization, thanks to its AI technology.
Turning Electronic Medical Records into Risk Predictors: Patient medical records are a gold mine of data, but sorting them out and getting useful results are a task that would waste a lot of human time and effort. This is where the power of edge computing and AI comes in.
Improving Existing Processes by Providing Robotic Support
Like every institution, the healthcare sector is also prone to resource and time leakage. This can eventually result in various inefficiencies which stack up with a scale to huge amounts. An example of this can be mislabeled data that comes from radiology and histopathology diagnoses. These are usually associated with human errors as the specialist has done his best; however, the mistake is later on verified by the doctor. The best options can be to repeat the diagnosis process or ask for an update with the lab. All this involves a great deal of time.
A medical tool makes use of the Edge-AI system and aims in handling such issues maintaining focus over microscopic slides used in histopathology.
Robot-assisted surgery: Surgical robots use Edge-AI to use information from previous operations to improve surgical techniques. Data from preoperative records are integrated with operational measures to improve the outcome of the operation. These operations are minimally invasive and the precision of the robot-assisted instrument helps reduce the degree of post-op trauma.
Autonomous robotic surgeries: Although currently limited to science fiction, robotic operations may become a reality in the future. Using machine learning to combine motor pattern recognition and visual interpretation of data can extend surgeon’s dexterity to robots and make autonomous robotic surgery a reality. Robotic surgery is currently limited to the remote control of robots by the surgeon via a computer, but this could change in the future [19].
Auxiliary Robots: These are robots that find application in various fields, including patient care, nursing, and the care of the elderly and debilitated patients.
Chatbots: Chatbots are AI-powered algorithms capable of conducting conversations with patients. They have the potential to become the first point of contact for primary health care. The severity of the request is determined and chatbots can either fix the problem or pass it on to the doctor. The widespread use of chatbots considerably reduces the workload of the doctor and avoids having to go unnecessarily to health professionals. This is the case of the company Calmedica, which uses artificial intelligence to communicate by SMS with patients before and after a consultation and to improve their follow-up [20]
Edge Analytics in Healthcare Powers Improved Patient Outcomes
Edge analytics can help physicians take a more holistic approach to disease management, better coordinate care journeys, and ultimately help patients stick to their long-term treatment better. Technology also plays a vital role in delivering care through telemedicine and remote patient monitoring.
Here are Some of its Applications
Audio-to-Text Transcripts: Healthcare professionals spend a lot of time entering medical notes into patients’ medical records. Voice-to-text transcription of these notes using AI would increase the time spent on patient care.
Precision Medicine: Making relevant patient data available to physicians is a further step in the development of precision medicine. It allows physicians to make medical decisions tailored to each patient and create treatment plans specific to each.
Edge-AI has the ability to analyze a large selection of patient data (symptoms, lifestyle, treatments, etc.) in order to offer a very precise and reliable diagnosis. In this context, one of the major challenges is to guarantee the interoperability of the different data sources (medical files, connected objects, applications, etc.) in order to offer complete analyzes.
Applications of Edge-AI in the Diagnosis of Diseases
Diagnosis using x-rays: The use of edge computing and AI to analyze x-ray images obtained by MRI machines, scanners, and x-rays have not only made it possible to obtain a diagnosis comparable to that of a radiologist, but the results were also much faster [22]. The use of AI in diagnosis is meant to be an adjunct for the radiologist, who can use AI for routine cases and use his resources for more complicated cases.
For example, the start-up Incepto uses artificial intelligence in medical imaging, with the aim of rapidly detecting cancer or cardiovascular and neurodegenerative diseases. It allows doctors to cope with the exponential increase in health data to be analyzed.
Use of AI in Oncology: AI-infused edge computing can effectively predict tumor behavior by combining analysis of clinical, microscopic, and molecular data. This would allow doctors to better understand the behavior of tumors as a whole, to better define their aggressiveness, and thus to select the treatment that would give the best results [21].
Edge-AI Enabled Imaging
In the recent few years, Edge-AI has expanded at a drastic rate particularly in the fields of medical imaging and diagnostics. This way, these technologies have helped medical researchers as well as healthcare providers to offer clinical practice which is not only effective but, flawless as well [23]. Making way for standardization and quantification, deep learning assists in the prevention of errors that may occur in the process of diagnostics along with improving upon the outcomes of the test. In addition to this, AI in edge technology is improving assessments of medical imaging so as to detect cases of Diabetic Retinopathy (DR) and malignancy. It also helps in quantifying the flow of blood in the form of visuals.
As per a recent poll carried out by European Radiology Experimental, more than 50% of healthcare leaders across the globe consider the role of AI in edge technology to be promising in the process of diagnosing and monitoring medical images and diagnostics and this percentage will grow significantly.
Remote Care
Another promising area that is clearly associated with the combination of two cutting edge technologies – edge computing and AI is reliable remote healthcare. Developing a remote patient monitoring system that will allow access to health data through connecting various medical as well as non-medical devices can be only possible by the combination of the two technologies. The platform is designed to offer patient care services, patient health programs, and chronic disease management. in addition to this, it also enables continuous care of the patients and the elderly at home which will reduce their cost of treatment.
The benefits from edge computing-based remote care will be highly significant. A study carried out in 2015 indicates an almost 50 percent reduction in 30-day readmissions and up to a 19 percent reduction in the 180-day readmission amongst patients who received remote care. The bottom line stands to benefit as well with estimates suggesting that telemedicine alone could help cut U.S. employer healthcare costs by as much as $6 billion annually.
Key AI Technologies that has Transformed the Healthcare Sector
Technologies like Deep Learning, NLP, Intelligent Robotics, and Context-Aware Processing are considered to be the backbone of Edge-AI in the real world. These are the technologies that have transformed the healthcare sector to a greater extent.
Deep Learning
The process of diagnosing and treating diseases is more likely to improve by the implementation of AI within the healthcare sector. Deep Learning is an essential component in Edge-AI and can be used for analyzing medical images and data so as to increase the potential of physicians for giving effective treatment to patients. Through deep learning, visually challenged can develop a sense of their environment as the AI-computer vision and the text-to-speech apps will describe the text, detect facial cues of the people nearby, assess the surroundings, and give an explanation of the environment. Within the revolution of deep learning, three trends include powerful Graphics Processing Units (GPUs), sophisticated neural network algorithms modeled on the human brain, and access to a huge amount of data from the web [24].
Natural Language Processing (NLP)
Edge-AI also has a very important role to play in converting a whole lot of complex data within meaningful and simple information and this process is made easier through NLP. The focus of NLP is to mimic responses that are similar to human responses through using algorithms for responding to queries and conducting conversations. In the healthcare sector, NLP is used for summarizing long narrative text like clinical notes or academic journals through identifying key phrases or concepts. Additionally, NLP can also map out data elements within EHR that are present in the form of unstructured texts and transforming them into structured data which becomes meaningful and helps in improving clinical decision making [25].
Intelligent Robotics
Edge-AI is an integral component in robotics. Robots have the potential to revolutionize life-caring facilities by reducing the need for hospitalization and helping people to stay healthy. AI in alliance with advanced humanoid design will enable robots to carry out social interactions and conversations with elderly patients so as to keep the presence of mind intact. As robots can be integrated with a higher level of flexibility and reach, they can be easily used for smaller incisions with a lot of precision within the infected area. Additionally, robots can also be used as a social partner for treating mental health issues of patients [26].
Context-Aware Processing
Other applications of Edge-AI in the healthcare sector are virtual assistant applications like Google Assistant, Apple Siri, Microsoft Cortana, and Amazon Alexa in the medical sector which will carry out tasks as has been commanded by the programmer. AI chatbots, when applied in the healthcare department will significantly reduce the burden over healthcare providers to coordinate care as well as to detect issues and diagnose any health-related concerns. When speaking of the evolution of health assistants, bots are rendered to be the greatest invention up till now. However, chatbots are only enablers within this process to help patients in getting to the right physician for their treatment.
Read More:
The Effectiveness Of Using The 4MAT Model
Case Study
AI and Edge Computing – Histopathology Analysis at Nvidia Jetson
Microscopic slides can be defective in a variety of ways. The mistake can occur at the staining procedure was performed to make tissues clear or; it can be the improper sample collection process, any issues with the sample itself, or the sample have been marked by the pathologist with a pen.
These aspects tend to reduce the reliability of the diagnoses made both by humans and analysis by AI models.
The technology was provided from the Virtum platform which is a comprehensive toolset to assess and process large-scale images having focused on microscopic. Virtum, is a cloud-based tool however, some of its functions can be transferred to an edge computing device through making use of the Nvidia Jetson program.
About Nvidia Jetson Nano
Nvidia Jetson is a small operating system which is used for designing, developing and prototype embedded system for both IoT and edge healthcare devices identical. The device can be compared with solutions offered by Arduino or Raspberry Pi and is supported by Nvidia. The size of the computer can be compared with a coffee pack which means it can easily be made part of any setup or can be transported without any hassles. The technology is repeatable and there are no issues in adding the component anywhere.
Why Scan Quality Validation is Important?
Scans of histopathology require higher levels of proficiency to work with. However, despite the skills of people working, there are chances of multiple errors which can be visible in the slides.
- regions containing ink
- out of focus regions in the slide
- torn or folded regions
- tiling effect because of stitching
- missed out regions during scanning
The development of AI in the healthcare department involves digitalization as well as the development of accessible as well as trustworthy datasets. The occurrence of any mistake listed above can result in the ineffectiveness of Ai automation and algorithms. In addition to this, a non-perfect slide within the dataset can impose a significant impact on the effectiveness of the entire network.
This solution will eventually help physicians to deliver accurate diagnoses as it will provide them information that is accurate and at the right time. Automated quality control after scanning helps in preventing clutter within the database and gives a chance of redo to the scanner or take any other adequate action if and when possible.
Gains
The device will greatly assist in the work of histopathology workers who are annotating slides. The device can be easily added up to any existing setup so as to gain insights into daily work or building new datasets. If the same device is adjusted with additional technologies, it can also be effectively used in radiology or similar other tasks that require marking over larger images.
The solution of the platform is that it can bring in considerable synergies when applied in conjunction with edge computing. Building a collection of properly marked annotated slides is very important in developing AI-based tools or automating any image-related workflow.
Summary
The solution of Nvidia edge AI-based shows how implementing single-step automation can result in the saving of both time and resources. In addition to this, it also shows that AI is the go-to strategy in the healthcare sector particularly when the aim is to save with bandwidth along with preparing better input for cloud-based solutions.
The Challenges of Adopting Edge-AI
The adoption of Edge-AI in healthcare opens up a number of possibilities, but it also comes with a series of challenges.
The phenomenon called “black box”
In some cases, AI systems offer solutions that cannot be explained. This phenomenon is explained by the complexity of these algorithms which perform a very large number of micro-reasonings that, placed end to end, allow the machine to issue its diagnosis. However, these are so numerous that it is impossible for a human to understand them, hence the black box.
However, to be acceptable, the decisions of the algorithm must be able to be understood and therefore explained. But in the case of the black box, the number of micro-reasonings performed by the machine is such that it is not possible to understand them [26].
Complexity of stakeholders
All players in the healthcare sector, including patients, healthcare professionals, pharmaceutical companies, hospitals, insurance companies, are stakeholders in the adoption of AI. Resistance to technology at any level would lead to problems in integrating the technology as a whole. As with any new technology, there is an initial reluctance to adopt it in the marketplace, with healthcare facilities and users concerned about its application and safety [27].
Compliance with regulations
According to the report “Giving a meaning to artificial intelligence” by Deputy Cédric Villani published in March 2018, artificial intelligence “opens up new opportunities to innovate” with constant pharmacopeia “by building a diagnosis and a therapeutic strategy more suited to the need. of the patient, his environment and his way of life ”. Bringing hope, artificial intelligence also generates several legal challenges [28].
AI can only develop with very large volumes of data. In France, the collection and use of health data are subject to a number of laws such as the GDPR and the incorporation of AI is subject to the approval of organizations to ensure that standards are maintained [29]. The challenge for political and legal institutions will be to allow the development of the interoperability of information systems within a protective legislative framework and to find the right balance between the use, access, and security of health data. The question is not to produce more data but, above all, to pool those that already exist, and to facilitate access within an ethical and protective framework [30].
Need Help or Advice in Content Management or In Marketing Strategy:
Do you want more advice? Do you have good practices to share? Express yourself in the comments.
Do you want any help to write content and drive more traffic and boost conversions; get in touch through Contact our team?
Read More
Workplace Aggression | Scholarly | Academic
Trends in Electropolishing – Scholarly Presentation
Modern Public Administration – Theory and Practice
References
[1] A. Abdel latif, A. Mohamed, C. F. Chiasserini, M. Tlili, and A. Erbad, “Edge computing for smart health: Context-aware approaches, opportunities, and challenges, ”IEEE Network, vol. 33,no. 3, pp. 196–203, 2019.
[2] S. Rani, S. H. Ahmed, and S. C. Shah, “Smart health: a novel paradigm to control the chickungunya virus, ”IEEE Internet of Things Journal, vol. 6, no. 2, pp. 1306–1311, 2018
[3] Bierzynski K., Escobar A., Eberl M. 2017 Second International Conference on Fog and Mobile Edge Computing (FMEC) IEEE; 2017. Cloud, fog and edge: cooperation for the future?
[4] Azimi I., Anzanpour A., Rahmani A.M., Pahikkala T., Levorato M., Liljeberg P., Dutt N. HiCH: hierarchical fog-assisted computing architecture for healthcare IoT. ACM Trans. Embed. Comput. Syst. (TECS) 2017;16(5s):174.
[5] Chiang M., Zhang T. Fog and IoT: an overview of research opportunities. IEEE Internet Things J. Dec. 2016;3(6):854–864
[6] Ibrahim W.N.H., Selamat A., Krejcar O., CHAUDHRY J. New Trends in Intelligent Software Methodologies, Tools and Techniques. IOS Press; 2018. Recent advances on fog health – a systematic literature review.
[7] Uddin M.Z. A wearable sensor-based activity prediction system to facilitate edge computing in smart healthcare system. J. Parallel Distrib. Comput. 2019;123:46–53
[8] Hartmann M., Hashmi U.S., Imran A. Edge computing in smart health care systems: review, challenges, and research directions. Trans. Emerg. Telecommun. Technol. 2019;e3710
[9] Azimi I., Takalo-Mattila J., Anzanpour A., Rahmani A.M., Soininen J.P., Liljeberg P. 2018 IEEE/ACM International Conference on Connected Health: Applications, Systems and Engineering Technologies (CHASE) IEEE; 2018. Empowering healthcare IoT systems with hierarchical edge-based deep learning; pp. 63–68.
[10] J. Azar, A. Makhoul, M. Barhamgi, and R. Couturier, “An energyefficient iot data compression approach for edge machine learn-ing,”Future Generation Computer Systems, vol. 96, 2019.
[11] Abdellatif A.A., Mohamed A., Chiasserini C.F., Tlili M., Erbad A. Edge computing for smart health: context-aware approaches, opportunities, and challenges. IEEE Netw. 2019;33(3):196–203
[12] Bishop,C.M.(2013)Model-basedmachinelearning. Philos.Trans.AMath.Phys.Eng.Sci. 371http://dx.doi.org/10.1098/rsta.2012.0222
[13] Ahmed Abdulkadir Ibrahim, Yasin Muhamma, Wang Zhuopeng,” “ IOT Patient Health Monitoring System” Int. Journal of Engineering Research and Application ISSN: 2248-9622, Vol. 7, Issue 10, October 2017, pp.01-03
[14] Musa, M. Opinion: Rise of the Robot Radiologists.Science. 2018. Available online: https://www.the-scientist.com/news-opinion/opinion–rise-of-the-robot-radiologists-64356 (accessed on 15 November 2020).
[15] Palanica, A.; Flaschner, P.; Thommandram, A.; Li, M.; Fossat, Y. Physicians’ Perceptions of Chatbots in Health Care: Cross-sectionalWeb-based Survey.J. Med Internet Res.2019,21, e12887
[16] Rohini Raina, Dr. Naveen Kumar Gondhi, “Machine Learning Techniques in IoT” International Journal of Scientific Research in Computer Science, Engineering and Information Technology © 2018 IJSRCSEIT | Volume 4 | Issue 1 | ISSN: 2456-3307
[17] A.Al-Fuqaha, M. Guizani, M. Mohammadi, M. Aledhari and M. Ayyash, “Enabling Technology for the internet of Health Things”. IEEE Translation and content mining, vol. 17, pp. 2347 – 2376, 2015
[18] Shubham Banka, Isha Madan and S.S. Saranya “Smart Healthcare Monitoring using IoT” International Journal of Applied Engineering Research ISSN 0973-4562 Volume 13, Number 15 (2018) pp. 11984-11989
[19] Yoon, S.; Lee, D. Artificial Intelligence and Robots in Healthcare: What are the Success Factors for Technology-based ServiceEncounters?Int. J. Healthc. Manag.2019,12, 218–225
[20] Ramesh, A.; Kambhampati, C.; Monson, J.; Drew, P. Artificial Intelligence in Medicine.Ann. R. Coll. Surg. Engl.2004,86, 334–338.
[21 Lee, S.; Lim, S.Living Innovation: From Value Creation to the Greater Good; Emerald Publishing Limited: Bingley, UK, 2018.
[22] Safavi, K.; Kalis, B. How AI can Change the Future of Health Care.Harv. Bus. Rev.2019. Available online: https://hbr.org/webinar/2019/02/how-ai-can-change-the-future-of-health-care (accessed on 15 June 2020).
[23] Magaña Espinoza P., Aquino-Santos R., Cárdenas-Benitez N., Aguilar-Velasco J., Buenrostro-Segura C., Edwards-Block A. WiSPH: a wireless sensor network-based home care monitoring system. Sensors. 2014;14(4):7096–7119
[24] LeCun, Y.; Bengio, Y.; Hinton, G. Deep Learning.Nature2015,251, 434–444.
[25] Sulleyman, A. Stephen Hawking Warns Artificial Intelligence ‘May Replace Humans Altogether’. 2017. Available online:https://www.independent.co.uk/life-style/gadgets-and-tech/news/stephen-hawking-artificial-intelligence-fears-ai-will-replace-humans-virus-life-a8034341.html (accessed on 15 October 2020).
[26] Capobianco G, Di Giacomo U, Martinelli F, et al. Wearable Devices for Human Activity Recognition and User Detection[C]//2019 27th Euromicro International Conference on Parallel, Distributed and Network-Based Processing (PDP). IEEE, 2019: 365-372
[27] Yoon; Lee, D. Effects of Key Value Co-creation Elements in the Healthcare System: Focusing on Technology Applications.Serv. Bus.2019,13, 389–417
[28] Rigby, M. Ethical Dimensions of Using Artificial Intelligence in Healthcare.AMA J. Ethics2019,21, E121–E124
[29] Lupton, M. Some Ethical and Legal Consequences of the Application of Artificial Intelligence in the Field of Medicine.TrendsMed.2018,18, 1–7.
[30] Luxton, D. Artificial Intelligence in Psychological Practice: Current and Future Applications and Implications.Prof. Psychol. Res.Pract.2014,45, 332–339
Comments are closed.